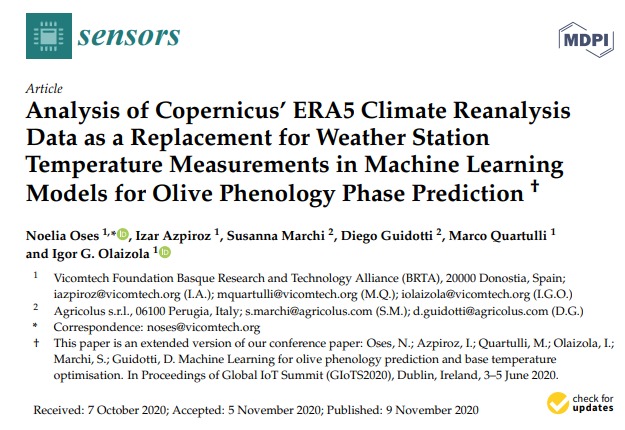
The full paper titled ‘Analysis of Copernicus’ ERA5 Climate Reanalysis Data as a Replacement for Weather Station Temperature Measurements in Machine Learning Models for Olive Phenology Phase Prediction’ was published in Sensors, a leading peer-reviewed open access journal on the science and technology of sensors. This paper, written by Noelia Oses, Izar Azpiroz, Marco Quartulli and Igor García of Vicomtech and Susanna Marchi and Diego Guidotti of Agricolus, is an extended version of the conference paper presented at the virtual Global IoT Summit (GIoTS 2020) earlier this year.
The study is part of the DEMETER project and the pilot focusing on Decision Support System (DSS) to support modern olive tree growing aims to optimize irrigation and fertilisation in olive orchards, as well as integrated pest management, through an on-line platform (Agricolus OLIWES). The proposed platform integrates software, sensors and open data to provide farmers and technicians with complete and efficient assistance in the olive tree growing and olive oil production. The key factor for the effective development of this DSS is the use of machine learning to link local climate conditions with crop phenology predictions.
The paper concluded that the differences in predicting the phenological phases of olive trees between ERA5-driven models and those based on weather station data were negligible. The accuracy obtained from data reanalysis using machine learning algorithms allowed the researchers to improve the traditional approach for modelling olive tree phenology, generating reliable predictions. Because weather station data are not globally available, ERA5-derived data can be conveniently used as substitute for recorded data. This approach provides a valid alternative for modelling phenological events where weather station data are not available or where their network does not ensure a sufficient coverage of the interested area.
Download the paper here