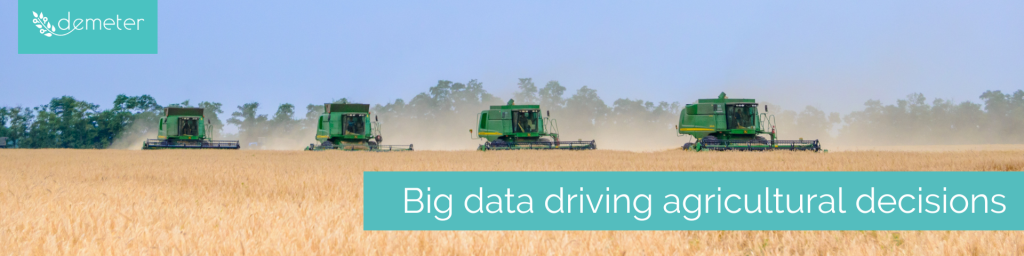
by Morteza Abdipour Chenarestansofla, John Deere, Intelligent Solution Group, Kaiserslautern, Germany
Bio
My name is Morteza Abdipour Chenarestansofla. I’m based in Germany and work as a product engineer at John Deere, European Technology & Innovation Center. I have +7 years’ experience with geospatial and satellite imagery data and +3 years industrial experience collaborating with farmers and working on machine telematics data and other sources of smart farming data in the field of crop farming. I’m currently a Ph.D. candidate in the field of agricultural science leading cluster 2 which involves 4 different pilots in DEMETER. I also function as John Deere representative “project coordinator” in DEMETER project.
I love to write about technology and how it contributes to pushing boundaries in science with a particular focus on data literacy, data culture, data interoperability, and AI in agrifood sectors.
Article
With the advancement of Precision Agriculture (PA) equipment, growers are now able to document field operations during the season. This leads to accumulation of a vast amount of data in terms of volume, verity, velocity, and dimensionality as the precision of sampling frequency is getting higher and higher. In addition, Smart Farming (SF) technologies such as drones, satellite imagery, and IoT add heterogeneity in the agricultural data pool. Hence big data is appearing rapidly in the field of agriculture faster than ever. Big data in agriculture enables farmers/advisers in making informed decisions and gain insights about the impact of their decisions on overall production system and sustainability. With all these data sources we ask ourselves how does data drive decisions in agriculture? To answer to that question, I would like to distinguish two major decision avenues in agriculture, operational decisions and agronomic decisions, that can make use of big data to drive agricultural decisions.
- Operational decisions
Operational decisions also refer to logistic decisions which include, but not limited to, the following:
- Planning an operation before actually going on the field
- Timing for performing an operation
- Time to complete an operation
- Predictive maintenance for vehicle health and downtime
- Emission reduction with optimizing operation execution
2. Agronomic decisions
Agronomic decisions,
- Spatial-temporal resource allocation
- Cost reduction
- Profit maximization
- Increase yield and support sustainability (Optimum yield production function)
- Reduce chemical substances applied on the field
John Deere supports farmers with the above-mentioned decisions by enabling farmers to document field operations throughout the season and integrating other data sources through collaboration with connected software companies to enhance agronomic decisions. One of the outstanding digital services offered by John Deere is a Farm Management Information System (FMIS) called John Deere Operation Center which is the John Deere cloud solution for telematics field-operations data management and analytics. The Operations Center is designed as an information hub, which makes data. It provides data access through a secure and anonymized fashion to the farmers and interesting parties with adequate access. The organization owner who has comprehensive access levels can add staff members into his/her organization and control their permission levels. Those members can be a third-party organization or company who wish to work with farmers’ data. Overall data access is managed through access rights, consent, and specific approval. See figure below.
The OpsCenter is a subscription free service that individual organizations with compatible John Deere PA equipment/hardware can gain benefits from. See figure below.
John Deere leverages machine learning technologies to make use of the existing data and enhance logistic operations.
John Deere supports EU funded projects by participating in research initiatives and its corporates farms. In one of our pilot projects within DEMETER called “in-service condition monitoring of agricultural machinery“, we focus on paving the way towards smarter machines that can follow existing/future EU emission regulation policies. This pilot intends on measuring critical engine parameters and raise awareness in case of malfunction and/or exceeding boundaries. With that data, the operator can take action before an adverse event occurs and, as a result, minimizing emissions produced by a defected engine. In this pilot we also integrate Data Quality (DQ) service from the DEMETER Enabler Hub via Agricultural Information Model (AIM). AIM solves the interoperability challenge between the pilot solution and the DQ enabler. AIM supplies a comprehensive data translation across different agrifood sectors and can be a worthwhile data model for application exchange and data exchange between different data spaces.